CDTM Research Stories: Resident Machine Learning Expert
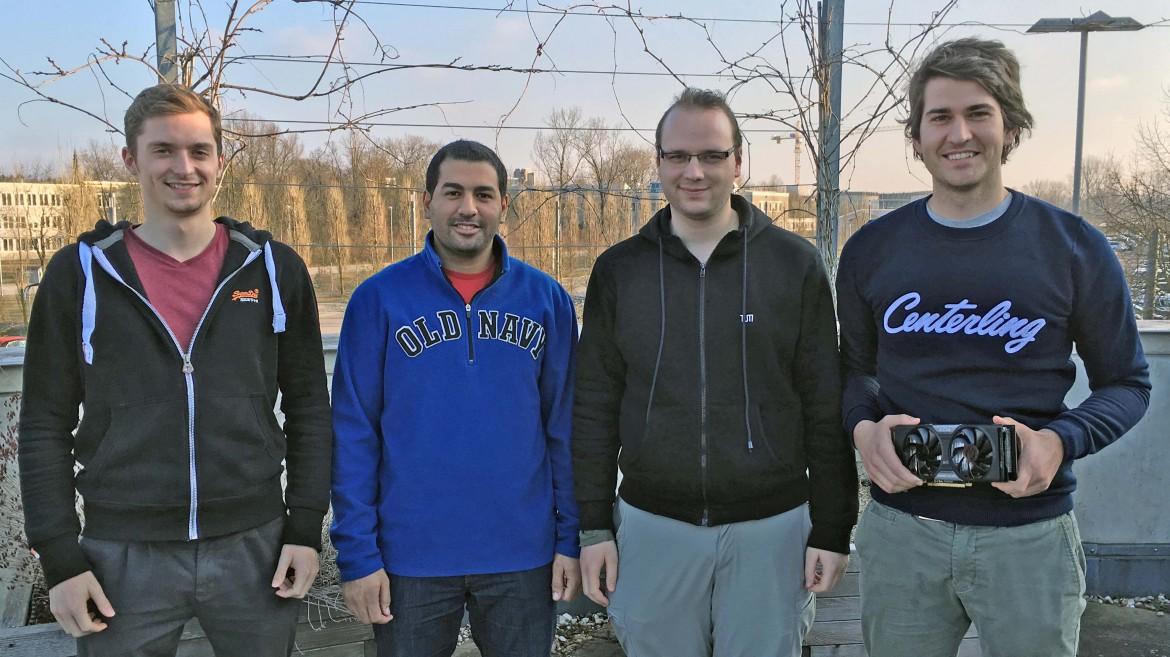
The resident expert in Machine Learning at CDTM is Center Assistant Patrick Christ. He was the one responsible for organizing and conducting the elective courses on Deep learning as well as Autonomous Drones. However his interest in Machine Learning is not just superficial as he is currently doing his doctorate in Deep Learning as well.
Patrick was recently the recipient of two major commercial research grants to help in his research: A $20,000 grant from AWS (Amazon Web Services) and a hardware grant from NVIDIA in the form of a 12 GB TITAN X GPU which is probably the fastest single GPU graphics card in the world at the moment. Both of these grants were awarded for his proposed work on automatic detection of cancer cells in livers employing image analysis using deep learning. Patrick plans to use this hardware to setup a deep learning cluster accelerated by GPU's to use in his research. That cluster as well as the grant money would also be available to students who are doing their masters research with him (there are four of them and more are welcome! )
Patrick recently talked about his research and work around Machine Learning at CDTM. One thing that many people want to know is where to start if they want to work in this field. Some consider it an applied mathematics and statistics field while others consider it as part of computer science. In reality however knowledge of both may not be sufficient and domain knowledge may also be required. Patrick, for example, said that he studied Physics at TUM with a focus on bio physics and gradually moved from hardware (sensors) while working with GE to data analysis and Machine Learning.
On a question about the reasons to choose CDTM for his PhD, Patrick talked about the freedom offered by CDTM as one can come up with their own research ideas and write their own research proposals along with CDTM board professor or supervisor. This is different from a university PhD where one can only apply for existing research project offered by the chair. Also PhD at CDTM is different in that it is more focused towards applications and thus has a rather broad scope and more breadth than depth. It is ultimately geared towards coming up with interdisciplinary research and projects.
Talking about his work, Patrick explained that he is working with medical data specifically liver images and trying to detect tumor cells in order to diagnose cancer at an early stage using image analysis. The dataset he is using is unlabelled and comprises of over ten thousand volumes of CT scans from five hundred patients. He is working with TUM's Chair of Image based Biomedical modeling with Prof. Dr. Bjoern Menze as well as with LMU's department of radiology. The goal of this research is a software product which can detect cancer timely and it thus falls squarely under the domain of preventive medicine.
One of the main costs of medical diagnostic imaging is the human resource costs and if part of the process can be aided with computer systems, the process can be made faster and cheaper and regular diagnostic scanning can become feasible. This in turn can go a long way towards achieving the goals of preventive medicine. This topic is so interesting that IBM is also developing a radiologists assistant powered by its AI platform Watson. If these systems keep on progressing as they have up till now, maybe in twenty years the role of Radiologists would also change from today and they would become more like specialized Data Scientists with these products and systems doing the grunt work for them. This research work thus has potential for being converted into such a commercial product and Patrick is indeed working with his Masters students to come up with business ideas around this technology.
Technically, Patricks and his team is trying to segment tumor cells using deep convolutional neural networks (CNNs) allowing voxel wise prediction of the tumor tissue. Whereas most research work in CNN is done on images, which are 2D, the novelty of this research comes from the fact that it is focusing instead on 3D imaging data and "volumes". This work involves adjusting existing methods used for 2D imaging for medical 3D volumes. 3D image data has some properties such as spatial locality which can be exploited. The CNN for volume data also do not use the usual basis functions such as those used in ImageNet Challenge but instead uses 3D convolutions.
Machine Learning and its applications are continuing to rise. As Prof Jürgen Schmidhuber, a renowned and leading Machine Learning expert, formerly associated with TUM, said that we are in a really interesting age to be working in AI because after so many years, AI has now finally come to a point where it can perform tasks better than humans and with higher accuracy. He is probably right considering Google's DeepMind system recently beat humans at GO, a game, which up to now was humans forte - unlike chess. Let's see how those advances will manifest themselves at CDTM with Patricks help and maybe (even probably) we would have a combined "Deep Learning Autonomous Drones" elective soon !